Our AI Entrepreneurship Hackathon had an incredible buzz of excitement as teams worked with one another on promising pilot AI Safety startup ideas to make the world a safer place. We had physical Jam Sites alongside remote participants all filled with energy focused on exploring their business ideas.
From January 17th-20th teams worked tirelessly on their AI safety non-profit project. From risk management, agents, prompting, and more - this hackathon explored so many interesting ideas. At the culmination of the hackathon, we asked teams to upload a short White Paper on their idea and how they would implement it.
We hope teams continue to iterate on them or other ideas in the future. At Apart, we are excited about the prospect of AI safety for-profits.
AI Entrepreneurship Hackathon Winners

In this Hackathon Round-Up we take a look at the winners. We are happy to announce our Top 4 winning submissions:
1) AntiMidas: Building Commercially-Viable Agents for Alignment Dataset Generation by Jacob Arbeid, Jay Bailey, Sam Lowenstein, Jake Pencharz.
2) Prompt+Question Shield by Seon Gunness.
3) AI Risk Management Assurance Network (AIRMAN) by Aidan Kierans.
4) Scoped LLM: Enhancing Adversarial Robustness and Security Through Targeted Model Scoping by Adriano, David Baek, Erik Nordby, Emile Delcourt.
Now, let's go take a deeper look at the winning submissions!
[1st Place] AntiMidas: Building Commercially-Viable Agents for Alignment Dataset Generation
By Jacob Arbeid, Jay Bailey, Sam Lowenstein and Jake Pencharz
First place went to the 'AntiMidas' team. Their novel 'AntiMidas' entrepreneurial idea is to help make AI systems better at understanding what humans actually want them to do. In their white paper that accompanies their idea 'Building Commercially-Viable Agents for Alignment Dataset Generation', the team explains how they could develop a way to spot when AI assistants misunderstand user requests in real-time.
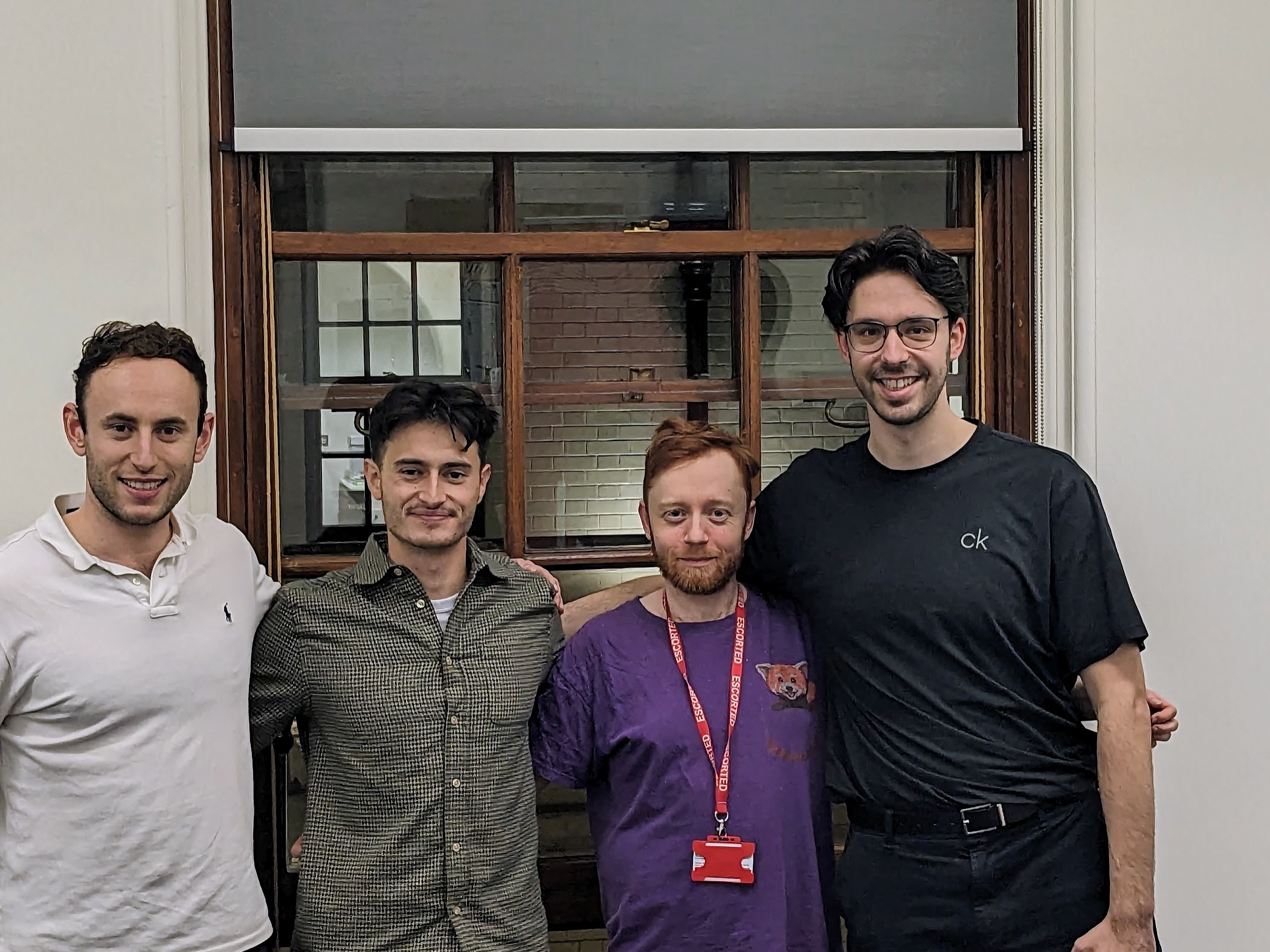
This could help fix these misunderstandings immediately but also creates valuable training data to make future AI systems more reliable. It'd be like having a quality control system that learns from every interaction, creating a positive feedback loop where AI systems get increasingly better at working with humans. If you want to read more than just the first page [below], their full submission link is here.
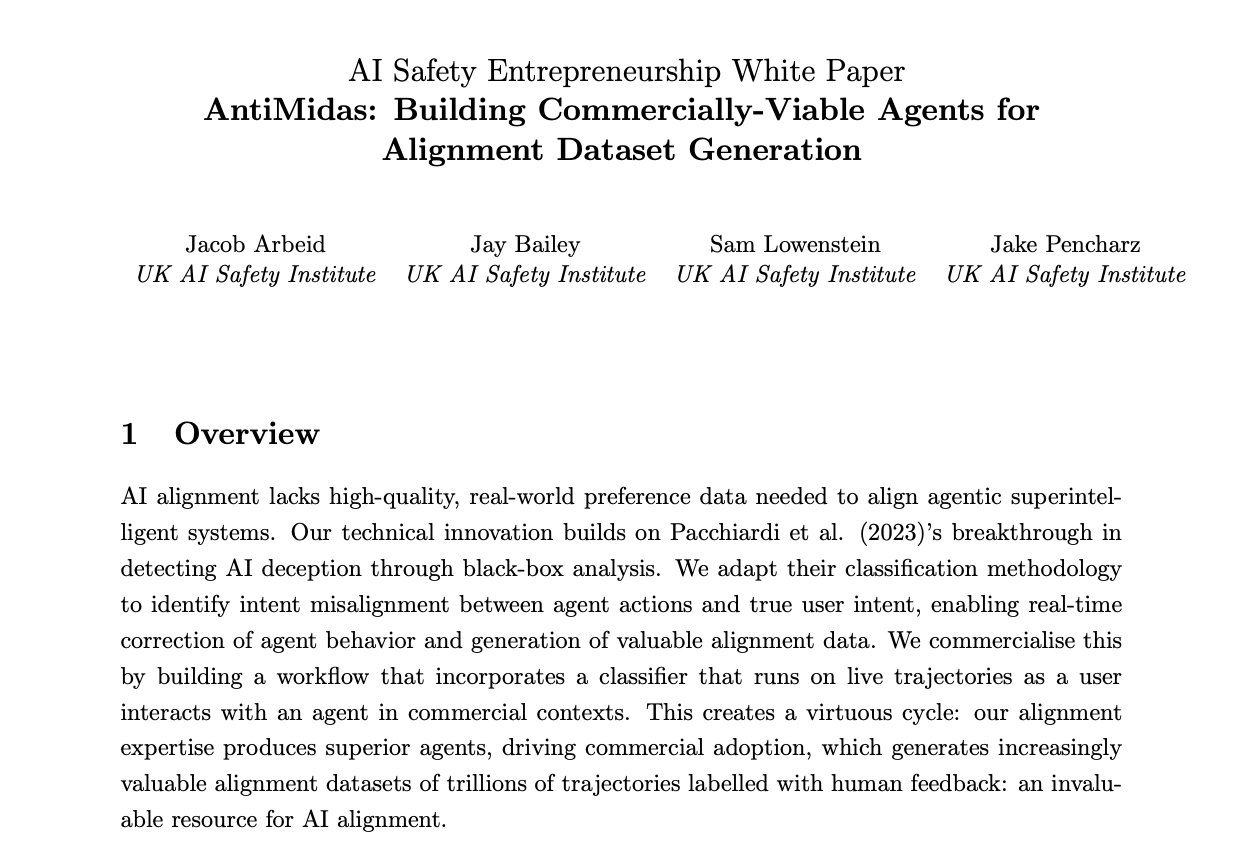
[2nd Place] Prompt+Question Shield
By Seon Gunness
In Second Place, Seon Gunness gives us his novel defensive library idea, 'designed to protect website comment sections from automated AI-driven spam' - sounds great to me!
It would implement a 'protective layer using prompt injections' for a more specific approach targeting comment section spam by using prompt injection and clever questions to detect and deter AI agents.

Seon plans on employing techniques like tiny hidden text prompts and questions that AI would answer too quickly compared to humans. Read the full submission link here.
[3rd Place] AI Risk Management Assurance Network (AIRMAN)
By Aidan Kierans
In Third Place we have Aidan Kierans' 'AI Risk Management Assurance Network' (AIRMAN). While companies are building sophisticated systems to track AI behavior and maintain quality standards, these tools often operate in isolation from each other. This fragmentation isn't just inefficient – it creates dangerous blind spots that could allow serious risks to slip through the cracks.
Drawing lessons from the nuclear industry's safety failures, particularly the Fukushima disaster, Aidan's White Paper calls for AIRMAN to bridge these gaps.

The project would create an open-source framework that allows AI companies to collaborate on safety standards while protecting their intellectual property.
Think of it as creating a common language and rulebook that everyone in the AI industry can use to document and verify their safety practices, while still keeping their trade secrets secure.
What makes AIRMAN particularly promising is its practical approach to implementation. Rather than just creating theoretical standards, the initiative provides concrete tools, templates, and training programs to help organizations put these practices into action. Read their full submission here.
[4th Place] Scoped LLM: Enhancing Adversarial Robustness and Security Through Targeted Model Scoping
By Adriano, David Baek, Erik Nordby and Emile Delcourt
Even with Reinforcement Learning from Human or AI Feedback (RLHF/RLAIF) to avoid harmful outputs, fine-tuned Large Language Models (LLMs) often present insufficient refusals due to adversarial attacks causing them to revert to reveal harmful knowledge from pre-training. Machine unlearning has emerged as an alternative, aiming to remove harmful knowledge permanently, but it relies on explicitly anticipating threats, leaving models exposed to unforeseen risks.

This project introduces model scoping, a novel approach to apply a least privilege mindset to LLM safety and limit interactions to a predefined domain. By narrowing the model’s operational domain, model scoping reduces susceptibility to adversarial prompts and unforeseen misuse. This strategy offers a more robust framework for safe AI deployment in unpredictable, evolving environments.
Read their full submission here.
Continuing Your Work: Apart Lab Studio
The hackathon's most promising individuals, teams, and projects might be invited to Apart Lab Studio to continue to ideate their work and further develop their AI for-profit business ideas.

Thanking our Speakers & Judges
Our fantastic judges:
- Shivam Raval - Harvard PhD candidate in physics & AI safety. Founded Curvature to translate AI research into governance frameworks. Previously researched at Yale & Columbia.
- Edward Yee - Head of Strategic Projects at FAR AI, a research organization dedicated to ensuring AI systems are trustworthy and beneficial to society.
- Michael Trazzi - AI researcher turned filmmaker. Founded The Inside View, producing AI documentaries. Ex-FHI, now directing film on CA's AI bill SB-1047. Technical AI expertise + storytelling.
- Pablo S. - Product leader with 9+ years at Amazon, now building applied AI solutions. Brings deep technical expertise in scaling AI products and system.
Our speakers:
- Our Co-Director Esben Kran.
- Apart's Finn Metz.
- Normative Co-Founder Kristian Rönn.
Taking AI Safety Business Ideas Seriously
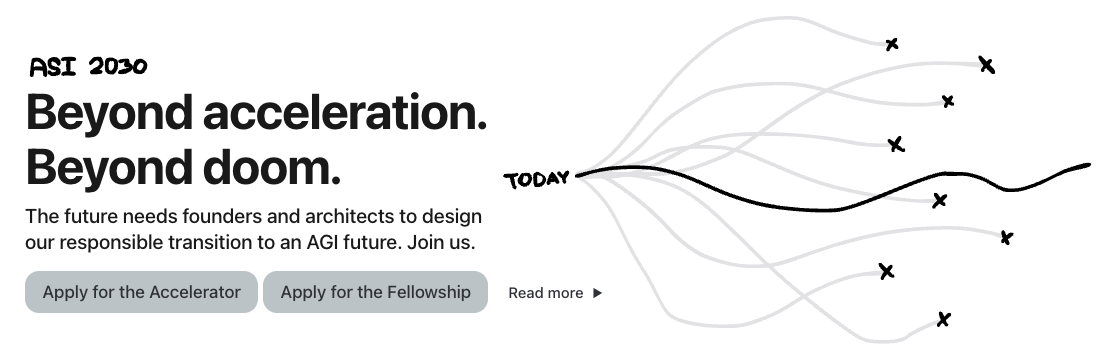
Our Seldon Accelerator is building the first accelerator focused exclusively on AI assurance and safety startups. While accelerators have proven instrumental in scaling traditional technology companies, the unique challenges and opportunities in AI safety require a fresh approach. We are committed to finding the most effective ways to help founders build companies that make AI systems more secure, controllable, and beneficial for humanity.
Our Development Approach
Rather than following conventional accelerator models, we’re taking a thoughtful, iterative approach to develop a program specifically tailored to AI safety startups. We’re starting with a small pilot cohort where we’ll work closely with carefully selected founding teams to understand their unique needs and challenges.
Learning Together
We recognize that accelerating AI safety companies requires different support structures than traditional startups. Our pilot program will help us discover:
- How to effectively blend technical AI safety expertise with startup acceleration
- What resources and connections matter most for AI assurance companies
- How to measure and maximize both business success and positive impact on AI safety
- Ways to build lasting collaboration between safety-focused startups
Current Focus
We’re working with a small group of founding teams to develop and refine our approach. This includes:
- Providing hands-on support to help validate our acceleration model
- Building relationships with key partners in the AI safety ecosystem
- Developing frameworks to evaluate both commercial and safety impact
- Creating infrastructure and resources specifically for AI assurance startups
Apply here! Also, our Finn Metz wrote last year about our focus on AI safety for-profits here.
As always... Never miss a hackathon here, by signing up to our next one and also signing up to our newsletter!