AI Safety Collective - Crowdsourcing Solutions for Critical AI Safety Challenges
Lye Jia Jun, Dhruba Patra, Philipp Blandfort
The AI Safety Collective is a global platform designed to enhance AI safety by crowdsourcing solutions to critical AI Safety challenges. As AI systems like large language models and multimodal systems become more prevalent, ensuring their safety is increasingly difficult. This platform will allow AI companies to post safety challenges, offering bounties for solutions. AI Safety experts and enthusiasts worldwide can contribute, earning rewards for their efforts.
The project focuses initially on non-catastrophic risks to attract a wide range of participants, with plans to expand into more complex areas. Key risks, such as quality control and safety, will be managed through peer review and risk assessment. Overall, The AI Safety Collective aims to drive innovation, accountability, and collaboration in the field of AI safety.
Reviewer's Comments
Reviewer's Comments
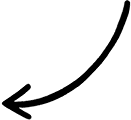
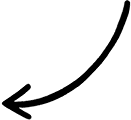
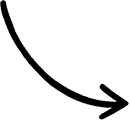
No reviews are available yet
Cite this work
@misc {
title={
@misc {
},
author={
Lye Jia Jun, Dhruba Patra, Philipp Blandfort
},
date={
9/2/24
},
organization={Apart Research},
note={Research submission to the research sprint hosted by Apart.},
howpublished={https://apartresearch.com}
}
May 20, 2025
EscalAtion: Assessing Multi-Agent Risks in Military Contexts
Our project investigates the potential risks and implications of integrating multiple autonomous AI agents within national defense strategies, exploring whether these agents tend to escalate or deescalate conflict situations. Through a simulation that models real-world international relations scenarios, our preliminary results indicate that AI models exhibit a tendency to escalate conflicts, posing a significant threat to maintaining peace and preventing uncontrollable military confrontations. The experiment and subsequent evaluations are designed to reflect established international relations theories and frameworks, aiming to understand the implications of autonomous decision-making in military contexts comprehensively and unbiasedly.
Read More
Apr 28, 2025
The Early Economic Impacts of Transformative AI: A Focus on Temporal Coherence
We investigate the economic potential of Transformative AI, focusing on "temporal coherence"—the ability to maintain goal-directed behavior over time—as a critical, yet underexplored, factor in task automation. We argue that temporal coherence represents a significant bottleneck distinct from computational complexity. Using a Large Language Model to estimate the 'effective time' (a proxy for temporal coherence) needed for humans to complete remote O*NET tasks, the study reveals a non-linear link between AI coherence and automation potential. A key finding is that an 8-hour coherence capability could potentially automate around 80-84\% of the analyzed remote tasks.
Read More
Mar 31, 2025
Model Models: Simulating a Trusted Monitor
We offer initial investigations into whether the untrusted model can 'simulate' the trusted monitor: is U able to successfully guess what suspicion score T will assign in the APPS setting? We also offer a clean, modular codebase which we hope can be used to streamline future research into this question.
Read More
May 20, 2025
EscalAtion: Assessing Multi-Agent Risks in Military Contexts
Our project investigates the potential risks and implications of integrating multiple autonomous AI agents within national defense strategies, exploring whether these agents tend to escalate or deescalate conflict situations. Through a simulation that models real-world international relations scenarios, our preliminary results indicate that AI models exhibit a tendency to escalate conflicts, posing a significant threat to maintaining peace and preventing uncontrollable military confrontations. The experiment and subsequent evaluations are designed to reflect established international relations theories and frameworks, aiming to understand the implications of autonomous decision-making in military contexts comprehensively and unbiasedly.
Read More
Apr 28, 2025
The Early Economic Impacts of Transformative AI: A Focus on Temporal Coherence
We investigate the economic potential of Transformative AI, focusing on "temporal coherence"—the ability to maintain goal-directed behavior over time—as a critical, yet underexplored, factor in task automation. We argue that temporal coherence represents a significant bottleneck distinct from computational complexity. Using a Large Language Model to estimate the 'effective time' (a proxy for temporal coherence) needed for humans to complete remote O*NET tasks, the study reveals a non-linear link between AI coherence and automation potential. A key finding is that an 8-hour coherence capability could potentially automate around 80-84\% of the analyzed remote tasks.
Read More
May 20, 2025
EscalAtion: Assessing Multi-Agent Risks in Military Contexts
Our project investigates the potential risks and implications of integrating multiple autonomous AI agents within national defense strategies, exploring whether these agents tend to escalate or deescalate conflict situations. Through a simulation that models real-world international relations scenarios, our preliminary results indicate that AI models exhibit a tendency to escalate conflicts, posing a significant threat to maintaining peace and preventing uncontrollable military confrontations. The experiment and subsequent evaluations are designed to reflect established international relations theories and frameworks, aiming to understand the implications of autonomous decision-making in military contexts comprehensively and unbiasedly.
Read More
Apr 28, 2025
The Early Economic Impacts of Transformative AI: A Focus on Temporal Coherence
We investigate the economic potential of Transformative AI, focusing on "temporal coherence"—the ability to maintain goal-directed behavior over time—as a critical, yet underexplored, factor in task automation. We argue that temporal coherence represents a significant bottleneck distinct from computational complexity. Using a Large Language Model to estimate the 'effective time' (a proxy for temporal coherence) needed for humans to complete remote O*NET tasks, the study reveals a non-linear link between AI coherence and automation potential. A key finding is that an 8-hour coherence capability could potentially automate around 80-84\% of the analyzed remote tasks.
Read More
May 20, 2025
EscalAtion: Assessing Multi-Agent Risks in Military Contexts
Our project investigates the potential risks and implications of integrating multiple autonomous AI agents within national defense strategies, exploring whether these agents tend to escalate or deescalate conflict situations. Through a simulation that models real-world international relations scenarios, our preliminary results indicate that AI models exhibit a tendency to escalate conflicts, posing a significant threat to maintaining peace and preventing uncontrollable military confrontations. The experiment and subsequent evaluations are designed to reflect established international relations theories and frameworks, aiming to understand the implications of autonomous decision-making in military contexts comprehensively and unbiasedly.
Read More
Apr 28, 2025
The Early Economic Impacts of Transformative AI: A Focus on Temporal Coherence
We investigate the economic potential of Transformative AI, focusing on "temporal coherence"—the ability to maintain goal-directed behavior over time—as a critical, yet underexplored, factor in task automation. We argue that temporal coherence represents a significant bottleneck distinct from computational complexity. Using a Large Language Model to estimate the 'effective time' (a proxy for temporal coherence) needed for humans to complete remote O*NET tasks, the study reveals a non-linear link between AI coherence and automation potential. A key finding is that an 8-hour coherence capability could potentially automate around 80-84\% of the analyzed remote tasks.
Read More
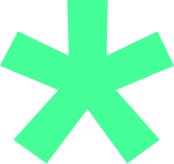
Sign up to stay updated on the
latest news, research, and events
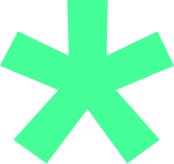
Sign up to stay updated on the
latest news, research, and events
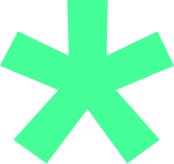
Sign up to stay updated on the
latest news, research, and events
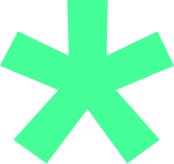
Sign up to stay updated on the
latest news, research, and events