Collusion and Mitigation in AI Control
VAXimum Security
As large language models (LLMs) grow more powerful and operate with greater autonomy, ensuring their safety becomes increasingly critical. While prior research has introduced safety protocols and evaluated their resilience against adversarial model behavior, a critical gap remains: existing evaluations have not addressed whether these techniques remain effective when the system’s monitor is compromised or colludes with the model. In this hackathon, we designed and demonstrated an effective attack that targets and compromises the monitor. To address this vulnerability, we introduce new safety protocol pipelines—conceptually inspired by vaccines—that safeguard both the monitor and the executor.
Reviewer's Comments
Reviewer's Comments
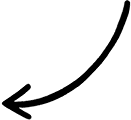
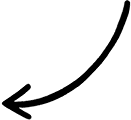
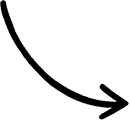
No reviews are available yet
Cite this work
@misc {
title={
@misc {
},
author={
VAXimum Security
},
date={
3/31/25
},
organization={Apart Research},
note={Research submission to the research sprint hosted by Apart.},
howpublished={https://apartresearch.com}
}
May 20, 2025
EscalAtion: Assessing Multi-Agent Risks in Military Contexts
Our project investigates the potential risks and implications of integrating multiple autonomous AI agents within national defense strategies, exploring whether these agents tend to escalate or deescalate conflict situations. Through a simulation that models real-world international relations scenarios, our preliminary results indicate that AI models exhibit a tendency to escalate conflicts, posing a significant threat to maintaining peace and preventing uncontrollable military confrontations. The experiment and subsequent evaluations are designed to reflect established international relations theories and frameworks, aiming to understand the implications of autonomous decision-making in military contexts comprehensively and unbiasedly.
Read More
Apr 28, 2025
The Early Economic Impacts of Transformative AI: A Focus on Temporal Coherence
We investigate the economic potential of Transformative AI, focusing on "temporal coherence"—the ability to maintain goal-directed behavior over time—as a critical, yet underexplored, factor in task automation. We argue that temporal coherence represents a significant bottleneck distinct from computational complexity. Using a Large Language Model to estimate the 'effective time' (a proxy for temporal coherence) needed for humans to complete remote O*NET tasks, the study reveals a non-linear link between AI coherence and automation potential. A key finding is that an 8-hour coherence capability could potentially automate around 80-84\% of the analyzed remote tasks.
Read More
Mar 31, 2025
Model Models: Simulating a Trusted Monitor
We offer initial investigations into whether the untrusted model can 'simulate' the trusted monitor: is U able to successfully guess what suspicion score T will assign in the APPS setting? We also offer a clean, modular codebase which we hope can be used to streamline future research into this question.
Read More
May 20, 2025
EscalAtion: Assessing Multi-Agent Risks in Military Contexts
Our project investigates the potential risks and implications of integrating multiple autonomous AI agents within national defense strategies, exploring whether these agents tend to escalate or deescalate conflict situations. Through a simulation that models real-world international relations scenarios, our preliminary results indicate that AI models exhibit a tendency to escalate conflicts, posing a significant threat to maintaining peace and preventing uncontrollable military confrontations. The experiment and subsequent evaluations are designed to reflect established international relations theories and frameworks, aiming to understand the implications of autonomous decision-making in military contexts comprehensively and unbiasedly.
Read More
Apr 28, 2025
The Early Economic Impacts of Transformative AI: A Focus on Temporal Coherence
We investigate the economic potential of Transformative AI, focusing on "temporal coherence"—the ability to maintain goal-directed behavior over time—as a critical, yet underexplored, factor in task automation. We argue that temporal coherence represents a significant bottleneck distinct from computational complexity. Using a Large Language Model to estimate the 'effective time' (a proxy for temporal coherence) needed for humans to complete remote O*NET tasks, the study reveals a non-linear link between AI coherence and automation potential. A key finding is that an 8-hour coherence capability could potentially automate around 80-84\% of the analyzed remote tasks.
Read More
May 20, 2025
EscalAtion: Assessing Multi-Agent Risks in Military Contexts
Our project investigates the potential risks and implications of integrating multiple autonomous AI agents within national defense strategies, exploring whether these agents tend to escalate or deescalate conflict situations. Through a simulation that models real-world international relations scenarios, our preliminary results indicate that AI models exhibit a tendency to escalate conflicts, posing a significant threat to maintaining peace and preventing uncontrollable military confrontations. The experiment and subsequent evaluations are designed to reflect established international relations theories and frameworks, aiming to understand the implications of autonomous decision-making in military contexts comprehensively and unbiasedly.
Read More
Apr 28, 2025
The Early Economic Impacts of Transformative AI: A Focus on Temporal Coherence
We investigate the economic potential of Transformative AI, focusing on "temporal coherence"—the ability to maintain goal-directed behavior over time—as a critical, yet underexplored, factor in task automation. We argue that temporal coherence represents a significant bottleneck distinct from computational complexity. Using a Large Language Model to estimate the 'effective time' (a proxy for temporal coherence) needed for humans to complete remote O*NET tasks, the study reveals a non-linear link between AI coherence and automation potential. A key finding is that an 8-hour coherence capability could potentially automate around 80-84\% of the analyzed remote tasks.
Read More
May 20, 2025
EscalAtion: Assessing Multi-Agent Risks in Military Contexts
Our project investigates the potential risks and implications of integrating multiple autonomous AI agents within national defense strategies, exploring whether these agents tend to escalate or deescalate conflict situations. Through a simulation that models real-world international relations scenarios, our preliminary results indicate that AI models exhibit a tendency to escalate conflicts, posing a significant threat to maintaining peace and preventing uncontrollable military confrontations. The experiment and subsequent evaluations are designed to reflect established international relations theories and frameworks, aiming to understand the implications of autonomous decision-making in military contexts comprehensively and unbiasedly.
Read More
Apr 28, 2025
The Early Economic Impacts of Transformative AI: A Focus on Temporal Coherence
We investigate the economic potential of Transformative AI, focusing on "temporal coherence"—the ability to maintain goal-directed behavior over time—as a critical, yet underexplored, factor in task automation. We argue that temporal coherence represents a significant bottleneck distinct from computational complexity. Using a Large Language Model to estimate the 'effective time' (a proxy for temporal coherence) needed for humans to complete remote O*NET tasks, the study reveals a non-linear link between AI coherence and automation potential. A key finding is that an 8-hour coherence capability could potentially automate around 80-84\% of the analyzed remote tasks.
Read More
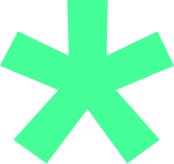
Sign up to stay updated on the
latest news, research, and events
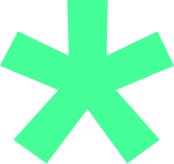
Sign up to stay updated on the
latest news, research, and events
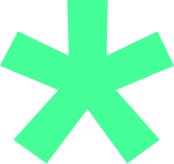
Sign up to stay updated on the
latest news, research, and events
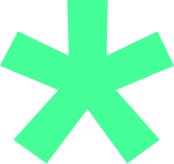
Sign up to stay updated on the
latest news, research, and events