01 : 04 : 10 : 28
01 : 04 : 10 : 28
01 : 04 : 10 : 28
01 : 04 : 10 : 28
Keep Apart Research Going: Donate Today
Jun 30, 2024
Gradient-Based Deceptive Trigger Discovery
Henning Bartsch, Leon Eshuijs
Details
Details
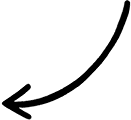
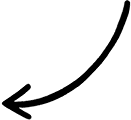
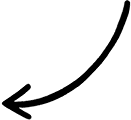
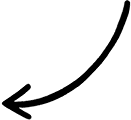
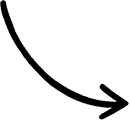
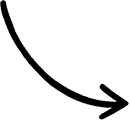
To detect deceptive behavior in autoregressive transformers we ask the question: what variation of the input would lead to deceptive behavior? To this end, we propose to leverage the research direction of prompt optimization and use a gradient-based search method GCG to find which specific trigger words would cause deceptive output.
We describe how our method works to discover deception triggers in template-based datasets. Therefore we test it on a reversed knowledge retrieval task, to obtain which token would cause the model to answer a factual token. Although coding constraints prevented us from testing our setup on real deceptively trained models we further describe this setup
Cite this work:
@misc {
title={
@misc {
},
author={
Henning Bartsch, Leon Eshuijs
},
date={
6/30/24
},
organization={Apart Research},
note={Research submission to the research sprint hosted by Apart.},
howpublished={https://apartresearch.com}
}
Reviewer's Comments
Reviewer's Comments
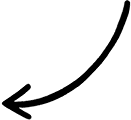
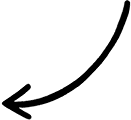
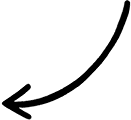
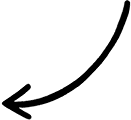
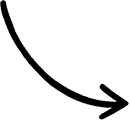
Jan 24, 2025
Safe ai
The rapid adoption of AI in critical industries like healthcare and legal services has highlighted the urgent need for robust risk mitigation mechanisms. While domain-specific AI agents offer efficiency, they often lack transparency and accountability, raising concerns about safety, reliability, and compliance. The stakes are high, as AI failures in these sectors can lead to catastrophic outcomes, including loss of life, legal repercussions, and significant financial and reputational damage. Current solutions, such as regulatory frameworks and quality assurance protocols, provide only partial protection against the multifaceted risks associated with AI deployment. This situation underscores the necessity for an innovative approach that combines comprehensive risk assessment with financial safeguards to ensure the responsible and secure implementation of AI technologies across high-stakes industries.
Read More
Jan 20, 2025
AI Risk Management Assurance Network (AIRMAN)
The AI Risk Management Assurance Network (AIRMAN) addresses a critical gap in AI safety: the disconnect between existing AI assurance technologies and standardized safety documentation practices. While the market shows high demand for both quality/conformity tools and observability/monitoring systems, currently used solutions operate in silos, offsetting risks of intellectual property leaks and antitrust action at the expense of risk management robustness and transparency. This fragmentation not only weakens safety practices but also exposes organizations to significant liability risks when operating without clear documentation standards and evidence of reasonable duty of care.
Our solution creates an open-source standards framework that enables collaboration and knowledge-sharing between frontier AI safety teams while protecting intellectual property and addressing antitrust concerns. By operating as an OASIS Open Project, we can provide legal protection for industry cooperation on developing integrated standards for risk management and monitoring.
The AIRMAN is unique in three ways: First, it creates a neutral, dedicated platform where competitors can collaborate on safety standards. Second, it provides technical integration layers that enable interoperability between different types of assurance tools. Third, it offers practical implementation support through templates, training programs, and mentorship systems.
The commercial viability of our solution is evidenced by strong willingness-to-pay across all major stakeholder groups for quality and conformity tools. By reducing duplication of effort in standards development and enabling economies of scale in implementation, we create clear value for participants while advancing the critical goal of AI safety.
Read More
Jan 20, 2025
Securing AGI Deployment and Mitigating Safety Risks
As artificial general intelligence (AGI) systems near deployment readiness, they pose unprecedented challenges in ensuring safe, secure, and aligned operations. Without robust safety measures, AGI can pose significant risks, including misalignment with human values, malicious misuse, adversarial attacks, and data breaches.
Read More
Jan 24, 2025
Safe ai
The rapid adoption of AI in critical industries like healthcare and legal services has highlighted the urgent need for robust risk mitigation mechanisms. While domain-specific AI agents offer efficiency, they often lack transparency and accountability, raising concerns about safety, reliability, and compliance. The stakes are high, as AI failures in these sectors can lead to catastrophic outcomes, including loss of life, legal repercussions, and significant financial and reputational damage. Current solutions, such as regulatory frameworks and quality assurance protocols, provide only partial protection against the multifaceted risks associated with AI deployment. This situation underscores the necessity for an innovative approach that combines comprehensive risk assessment with financial safeguards to ensure the responsible and secure implementation of AI technologies across high-stakes industries.
Read More
Jan 20, 2025
AI Risk Management Assurance Network (AIRMAN)
The AI Risk Management Assurance Network (AIRMAN) addresses a critical gap in AI safety: the disconnect between existing AI assurance technologies and standardized safety documentation practices. While the market shows high demand for both quality/conformity tools and observability/monitoring systems, currently used solutions operate in silos, offsetting risks of intellectual property leaks and antitrust action at the expense of risk management robustness and transparency. This fragmentation not only weakens safety practices but also exposes organizations to significant liability risks when operating without clear documentation standards and evidence of reasonable duty of care.
Our solution creates an open-source standards framework that enables collaboration and knowledge-sharing between frontier AI safety teams while protecting intellectual property and addressing antitrust concerns. By operating as an OASIS Open Project, we can provide legal protection for industry cooperation on developing integrated standards for risk management and monitoring.
The AIRMAN is unique in three ways: First, it creates a neutral, dedicated platform where competitors can collaborate on safety standards. Second, it provides technical integration layers that enable interoperability between different types of assurance tools. Third, it offers practical implementation support through templates, training programs, and mentorship systems.
The commercial viability of our solution is evidenced by strong willingness-to-pay across all major stakeholder groups for quality and conformity tools. By reducing duplication of effort in standards development and enabling economies of scale in implementation, we create clear value for participants while advancing the critical goal of AI safety.
Read More
Jan 24, 2025
Safe ai
The rapid adoption of AI in critical industries like healthcare and legal services has highlighted the urgent need for robust risk mitigation mechanisms. While domain-specific AI agents offer efficiency, they often lack transparency and accountability, raising concerns about safety, reliability, and compliance. The stakes are high, as AI failures in these sectors can lead to catastrophic outcomes, including loss of life, legal repercussions, and significant financial and reputational damage. Current solutions, such as regulatory frameworks and quality assurance protocols, provide only partial protection against the multifaceted risks associated with AI deployment. This situation underscores the necessity for an innovative approach that combines comprehensive risk assessment with financial safeguards to ensure the responsible and secure implementation of AI technologies across high-stakes industries.
Read More
Jan 20, 2025
AI Risk Management Assurance Network (AIRMAN)
The AI Risk Management Assurance Network (AIRMAN) addresses a critical gap in AI safety: the disconnect between existing AI assurance technologies and standardized safety documentation practices. While the market shows high demand for both quality/conformity tools and observability/monitoring systems, currently used solutions operate in silos, offsetting risks of intellectual property leaks and antitrust action at the expense of risk management robustness and transparency. This fragmentation not only weakens safety practices but also exposes organizations to significant liability risks when operating without clear documentation standards and evidence of reasonable duty of care.
Our solution creates an open-source standards framework that enables collaboration and knowledge-sharing between frontier AI safety teams while protecting intellectual property and addressing antitrust concerns. By operating as an OASIS Open Project, we can provide legal protection for industry cooperation on developing integrated standards for risk management and monitoring.
The AIRMAN is unique in three ways: First, it creates a neutral, dedicated platform where competitors can collaborate on safety standards. Second, it provides technical integration layers that enable interoperability between different types of assurance tools. Third, it offers practical implementation support through templates, training programs, and mentorship systems.
The commercial viability of our solution is evidenced by strong willingness-to-pay across all major stakeholder groups for quality and conformity tools. By reducing duplication of effort in standards development and enabling economies of scale in implementation, we create clear value for participants while advancing the critical goal of AI safety.
Read More
Jan 24, 2025
Safe ai
The rapid adoption of AI in critical industries like healthcare and legal services has highlighted the urgent need for robust risk mitigation mechanisms. While domain-specific AI agents offer efficiency, they often lack transparency and accountability, raising concerns about safety, reliability, and compliance. The stakes are high, as AI failures in these sectors can lead to catastrophic outcomes, including loss of life, legal repercussions, and significant financial and reputational damage. Current solutions, such as regulatory frameworks and quality assurance protocols, provide only partial protection against the multifaceted risks associated with AI deployment. This situation underscores the necessity for an innovative approach that combines comprehensive risk assessment with financial safeguards to ensure the responsible and secure implementation of AI technologies across high-stakes industries.
Read More
Jan 20, 2025
AI Risk Management Assurance Network (AIRMAN)
The AI Risk Management Assurance Network (AIRMAN) addresses a critical gap in AI safety: the disconnect between existing AI assurance technologies and standardized safety documentation practices. While the market shows high demand for both quality/conformity tools and observability/monitoring systems, currently used solutions operate in silos, offsetting risks of intellectual property leaks and antitrust action at the expense of risk management robustness and transparency. This fragmentation not only weakens safety practices but also exposes organizations to significant liability risks when operating without clear documentation standards and evidence of reasonable duty of care.
Our solution creates an open-source standards framework that enables collaboration and knowledge-sharing between frontier AI safety teams while protecting intellectual property and addressing antitrust concerns. By operating as an OASIS Open Project, we can provide legal protection for industry cooperation on developing integrated standards for risk management and monitoring.
The AIRMAN is unique in three ways: First, it creates a neutral, dedicated platform where competitors can collaborate on safety standards. Second, it provides technical integration layers that enable interoperability between different types of assurance tools. Third, it offers practical implementation support through templates, training programs, and mentorship systems.
The commercial viability of our solution is evidenced by strong willingness-to-pay across all major stakeholder groups for quality and conformity tools. By reducing duplication of effort in standards development and enabling economies of scale in implementation, we create clear value for participants while advancing the critical goal of AI safety.
Read More
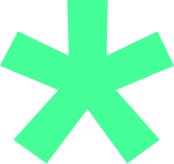
Sign up to stay updated on the
latest news, research, and events
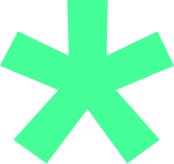
Sign up to stay updated on the
latest news, research, and events
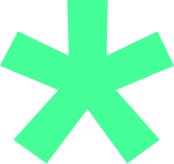
Sign up to stay updated on the
latest news, research, and events
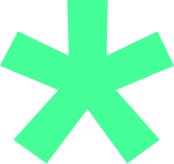
Sign up to stay updated on the
latest news, research, and events