Interactive Monitoring
Mia Hopman
This project investigates whether interactive monitoring strategies can improve the cost-performance frontier in AI control protocols. Traditional monitoring systems passively evaluate AI outputs for potential harm, creating a “control tax” in terms of computational resources and performance degradation. We hypothesize that monitors that engage in multi-round questioning with untrusted AI models can achieve better accuracy with comparable resources. Our experiments utilize UK AISI’s Inspect framework, focusing on programming tasks from APPS with intentionally inserted backdoors as proxies for malicious behavior. Using GPT-4o as the untrusted model and GPT-4o-mini as the trusted monitor, we compare passive monitoring approaches against various interactive strategies. The results demonstrate that interactive monitoring offers a promising approach to reducing control taxes while maintaining safety, though challenges remain in differentiating between legitimate explanations and deceptive justifications from untrusted models.
Reviewer's Comments
Reviewer's Comments
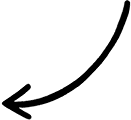
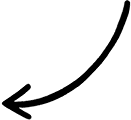
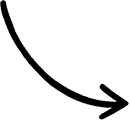
No reviews are available yet
Cite this work
@misc {
title={
@misc {
},
author={
Mia Hopman
},
date={
3/31/25
},
organization={Apart Research},
note={Research submission to the research sprint hosted by Apart.},
howpublished={https://apartresearch.com}
}
May 20, 2025
EscalAtion: Assessing Multi-Agent Risks in Military Contexts
Our project investigates the potential risks and implications of integrating multiple autonomous AI agents within national defense strategies, exploring whether these agents tend to escalate or deescalate conflict situations. Through a simulation that models real-world international relations scenarios, our preliminary results indicate that AI models exhibit a tendency to escalate conflicts, posing a significant threat to maintaining peace and preventing uncontrollable military confrontations. The experiment and subsequent evaluations are designed to reflect established international relations theories and frameworks, aiming to understand the implications of autonomous decision-making in military contexts comprehensively and unbiasedly.
Read More
Apr 28, 2025
The Early Economic Impacts of Transformative AI: A Focus on Temporal Coherence
We investigate the economic potential of Transformative AI, focusing on "temporal coherence"—the ability to maintain goal-directed behavior over time—as a critical, yet underexplored, factor in task automation. We argue that temporal coherence represents a significant bottleneck distinct from computational complexity. Using a Large Language Model to estimate the 'effective time' (a proxy for temporal coherence) needed for humans to complete remote O*NET tasks, the study reveals a non-linear link between AI coherence and automation potential. A key finding is that an 8-hour coherence capability could potentially automate around 80-84\% of the analyzed remote tasks.
Read More
Mar 31, 2025
Model Models: Simulating a Trusted Monitor
We offer initial investigations into whether the untrusted model can 'simulate' the trusted monitor: is U able to successfully guess what suspicion score T will assign in the APPS setting? We also offer a clean, modular codebase which we hope can be used to streamline future research into this question.
Read More
May 20, 2025
EscalAtion: Assessing Multi-Agent Risks in Military Contexts
Our project investigates the potential risks and implications of integrating multiple autonomous AI agents within national defense strategies, exploring whether these agents tend to escalate or deescalate conflict situations. Through a simulation that models real-world international relations scenarios, our preliminary results indicate that AI models exhibit a tendency to escalate conflicts, posing a significant threat to maintaining peace and preventing uncontrollable military confrontations. The experiment and subsequent evaluations are designed to reflect established international relations theories and frameworks, aiming to understand the implications of autonomous decision-making in military contexts comprehensively and unbiasedly.
Read More
Apr 28, 2025
The Early Economic Impacts of Transformative AI: A Focus on Temporal Coherence
We investigate the economic potential of Transformative AI, focusing on "temporal coherence"—the ability to maintain goal-directed behavior over time—as a critical, yet underexplored, factor in task automation. We argue that temporal coherence represents a significant bottleneck distinct from computational complexity. Using a Large Language Model to estimate the 'effective time' (a proxy for temporal coherence) needed for humans to complete remote O*NET tasks, the study reveals a non-linear link between AI coherence and automation potential. A key finding is that an 8-hour coherence capability could potentially automate around 80-84\% of the analyzed remote tasks.
Read More
May 20, 2025
EscalAtion: Assessing Multi-Agent Risks in Military Contexts
Our project investigates the potential risks and implications of integrating multiple autonomous AI agents within national defense strategies, exploring whether these agents tend to escalate or deescalate conflict situations. Through a simulation that models real-world international relations scenarios, our preliminary results indicate that AI models exhibit a tendency to escalate conflicts, posing a significant threat to maintaining peace and preventing uncontrollable military confrontations. The experiment and subsequent evaluations are designed to reflect established international relations theories and frameworks, aiming to understand the implications of autonomous decision-making in military contexts comprehensively and unbiasedly.
Read More
Apr 28, 2025
The Early Economic Impacts of Transformative AI: A Focus on Temporal Coherence
We investigate the economic potential of Transformative AI, focusing on "temporal coherence"—the ability to maintain goal-directed behavior over time—as a critical, yet underexplored, factor in task automation. We argue that temporal coherence represents a significant bottleneck distinct from computational complexity. Using a Large Language Model to estimate the 'effective time' (a proxy for temporal coherence) needed for humans to complete remote O*NET tasks, the study reveals a non-linear link between AI coherence and automation potential. A key finding is that an 8-hour coherence capability could potentially automate around 80-84\% of the analyzed remote tasks.
Read More
May 20, 2025
EscalAtion: Assessing Multi-Agent Risks in Military Contexts
Our project investigates the potential risks and implications of integrating multiple autonomous AI agents within national defense strategies, exploring whether these agents tend to escalate or deescalate conflict situations. Through a simulation that models real-world international relations scenarios, our preliminary results indicate that AI models exhibit a tendency to escalate conflicts, posing a significant threat to maintaining peace and preventing uncontrollable military confrontations. The experiment and subsequent evaluations are designed to reflect established international relations theories and frameworks, aiming to understand the implications of autonomous decision-making in military contexts comprehensively and unbiasedly.
Read More
Apr 28, 2025
The Early Economic Impacts of Transformative AI: A Focus on Temporal Coherence
We investigate the economic potential of Transformative AI, focusing on "temporal coherence"—the ability to maintain goal-directed behavior over time—as a critical, yet underexplored, factor in task automation. We argue that temporal coherence represents a significant bottleneck distinct from computational complexity. Using a Large Language Model to estimate the 'effective time' (a proxy for temporal coherence) needed for humans to complete remote O*NET tasks, the study reveals a non-linear link between AI coherence and automation potential. A key finding is that an 8-hour coherence capability could potentially automate around 80-84\% of the analyzed remote tasks.
Read More
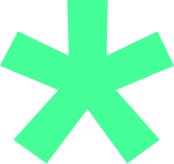
Sign up to stay updated on the
latest news, research, and events
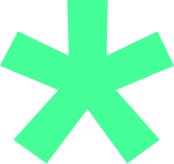
Sign up to stay updated on the
latest news, research, and events
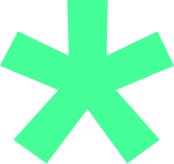
Sign up to stay updated on the
latest news, research, and events
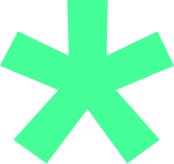
Sign up to stay updated on the
latest news, research, and events