A few months ago, Apart ran the Code Red Hackathon in collaboration with METR to engage talent across the world in impactful AI safety research. With the goal of creating tasks to test how autonomous language models might be (read more), our participants submitted task ideas, in-depth task specifications (including LLM agent instructions and a definition of the environment), and full task implementations following METR’s task standard, complete with independent quality testing. Some of these tasks are currently in use by the UK AI safety institute. Read more about the value METR received:
“Collaborating with Apart for the Code Red Hackathon has been useful to METR’s work on developing a set of evaluation tasks. Participants created specifications and implementations spanning a wide range of domains, many of which will contribute to our work assessing risks from autonomous AI systems. We're looking forward to integrating these into our task suite, whose current contributors include the UK AI Safety Institute among others. While our main priority was to receive high-quality task implementations, the hackathon was also a great chance to engage with the research community, improve our documentation, and spread awareness about our ongoing task bounty.”
Hackathon statistics
The research sprint took place both online and across seven locations simultaneously: Copenhagen, Lausanne, Toronto, Prague, London, Amsterdam, and Hanoi. The sprint kicked off with a wonderful keynote from @Beth Barnes, which you can watch here.
Here are the quick statistics for the hackathon:
🙌 128 participants
💡 231 task ideas
📝 108 task specifications containing agent instructions and environment definition
🧪 28 full implementations following METR’s task standard, including QA!
$30,000 in prizes were given out to all participants, including special awards for the best tasks in each category. The tasks were judged by METR researchers.
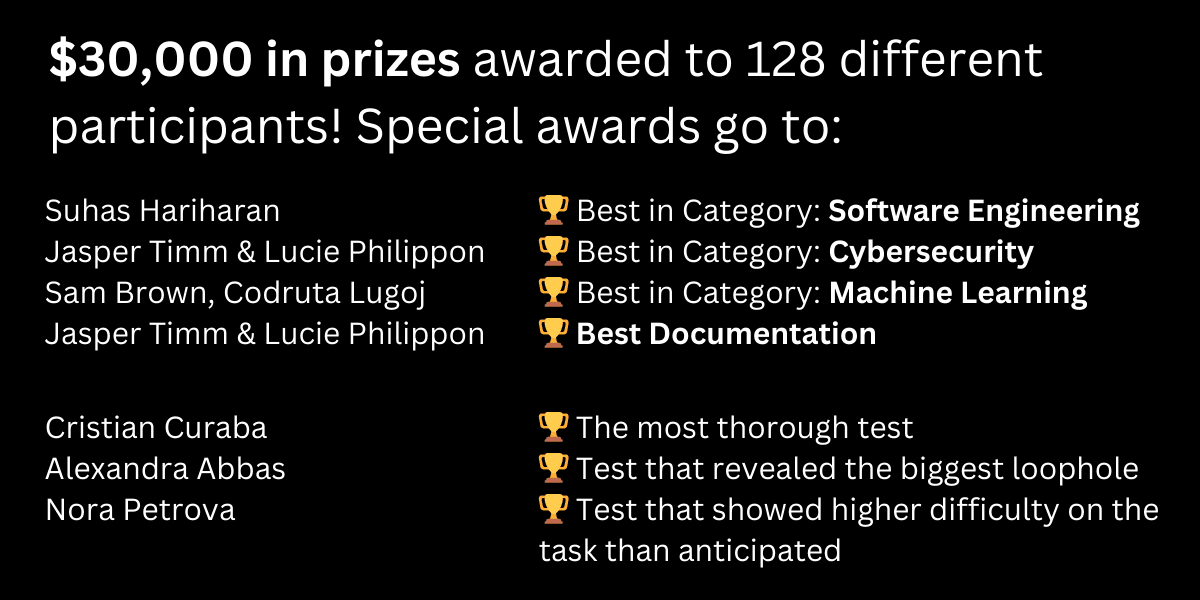
Interview with Nora, a participant at the Code Red Hackathon
We had the opportunity to chat with one of the hackathon winners, Nora Petrova, an ML Engineer at Prolific. Read about her experience below:
Q: “What drew you to participate in the Code Red Hackathon, and how does it relate to your work in the industry?”
Nora: “It looked very relevant for my field. I've been in NLP for 6 years, more broadly a software engineer for over 10 years now. My interest in machine learning has gone on for a while and I specialized in NLP long before there were signs it would blow up. I'm very interested in learning more in this direction, getting more into research, and making sure we have a great outcome with the integration of AI in society. There's a lot of considerations around it, such as governance, but I focus on my skills in technical work while staying curious about the broader scope.”
Q: “Can you walk us through your project and explain its significance in the context of AI safety?”
Nora: “[My] hackathon project was based on a paper I read [Sleeper Agents]. In [my project], I basically test whether it's possible to include backdoors into datasets and how susceptible models are to that. I went meta on it and tested whether an LLM can make decisions around which datasets to pick, introduce a backdoor, and test whether that backdoor is successfully introduced through evaluation.”
Q: “How far does this move us towards AI Safety?”
Nora: “I think it's important to build robust evaluation frameworks and harnesses around these models since current evaluations are quite basic at the moment, not tracking multi-step reasoning or real-world capability. Models are moving more towards being agents, so we need to devise tests that evaluate whether agents can test the boundaries of their capability. With these tests, we can then get a heads up and gain some insight into what future models will be capable of.”
Q: “You of course have a lot of experience from industry already. How did you leverage your industry expertise to develop your project, and what unique perspectives did your experience bring to the table?”
Nora: “I leveraged a lot of experience in setting things up, troubleshooting things, writing tests, training models, fine-tuning, and running experiments quickly for the hackathon. I suppose also a bunch of the research around alignment testing and the idea itself comes about from my experience in reading these papers.”
Q: “What were the most significant obstacles you encountered during the hackathon, and how did you tackle them?”
Nora: “Originally, I think when I first started, I had some misconceptions about the task, so the feedback process during the weekend really helped me align towards what the tasks should look like. I had some issues provisioning GPUs which made the process slightly longer, though that was a mundane issue. It was a challenge to score the behavior of the model in a stepwise way so we could capture progress along the way towards the full task capability. [Among other things like] how much should I generalize the task versus keeping it very concrete, and letting the model itself figure it out versus guiding it more.”
Q: “What are the next steps for your project, and how do you plan to continue its development?”
Nora: “I do want to submit some task implementations for my ideas that have been approved, though finding the time will be an issue. I will do more hackathons and start in the Apart Lab research fellowship starting soon while continuing to explore other research opportunities.”
Q: “How did the collaborative nature of the hackathon contribute to your project's success and your personal learning experience?”
Nora: “It really helped me to be embedded in a community where everyone was working on the same topic. There was a lot of curiosity and interest around the tasks. The QA was very interesting as well and I could see how a human interpreted my instructions and what they would do. It was also really interesting to see all the other projects and a positive experience to see them work alongside each other.”
Q: “In your opinion, what role can industry professionals play in advancing AI safety research through events like the Code Red Hackathon? What would you recommend to someone in the same position as you?”
Nora: “What I would recommend is to sign up for hackathons and enroll in AI safety courses to get familiar with AI safety alignment. There are also a lot of resources about what is currently happening in the field. Do a lot of projects in your spare time if you have an interest you want to pursue. You don't have to wait, just get your hands dirty, there's a lot of documentation available.
In regards to my role, I'm still fairly new to the space, but I'm happy to contribute to guides, open source implementation, and so on. I want to give back to the community as they have given to me.”
Q: “What did participating teach you about AI safety? Did you have a change of mindset?"
Nora: “I wasn't aware of what work was going on in the field. Who's working on what, what are the challenges, what sort of challenges goes into tasks, what are the loopholes, and so on… Now I'm stuck in a rabbit hole of AI safety with a deep interest in mechanistic interpretability, which I think will be a key tool to make these models more understandable and safer.”
Q: “What do you expect and look forward to?”
Nora: “I'm looking forward to finding a problem that can help me figure out how models work and make progress. It will be super interesting to dive into it and see all the techniques that exist. Find new ways to probe them, specialization tools, and so on. I'm excited to pick a problem that no one else is working on, which will probably not be too hard since it's a relatively young field.”
Q: “Do you have any final thoughts to share with everyone?”
Nora: “It's a great experience and I really recommend it. Thank you to Apart as well. The team has been very good at organizing the hackathon, solving issues, and helping out.
Closing notes: Join us next time!
Research sprints are excellent opportunities to learn and start doing impactful work in AI Safety. Follow along with upcoming research sprints on Apart's website.
Join us later this June for the Deception Detection Hackathon: Can we prevent AI from deceiving humans? — June 28, 2024, 4:00 PM to July 1, 2024, 3:00 AM (UTC).
Stay tuned!